Unlocking the Power of Image Annotation Tool Open Source
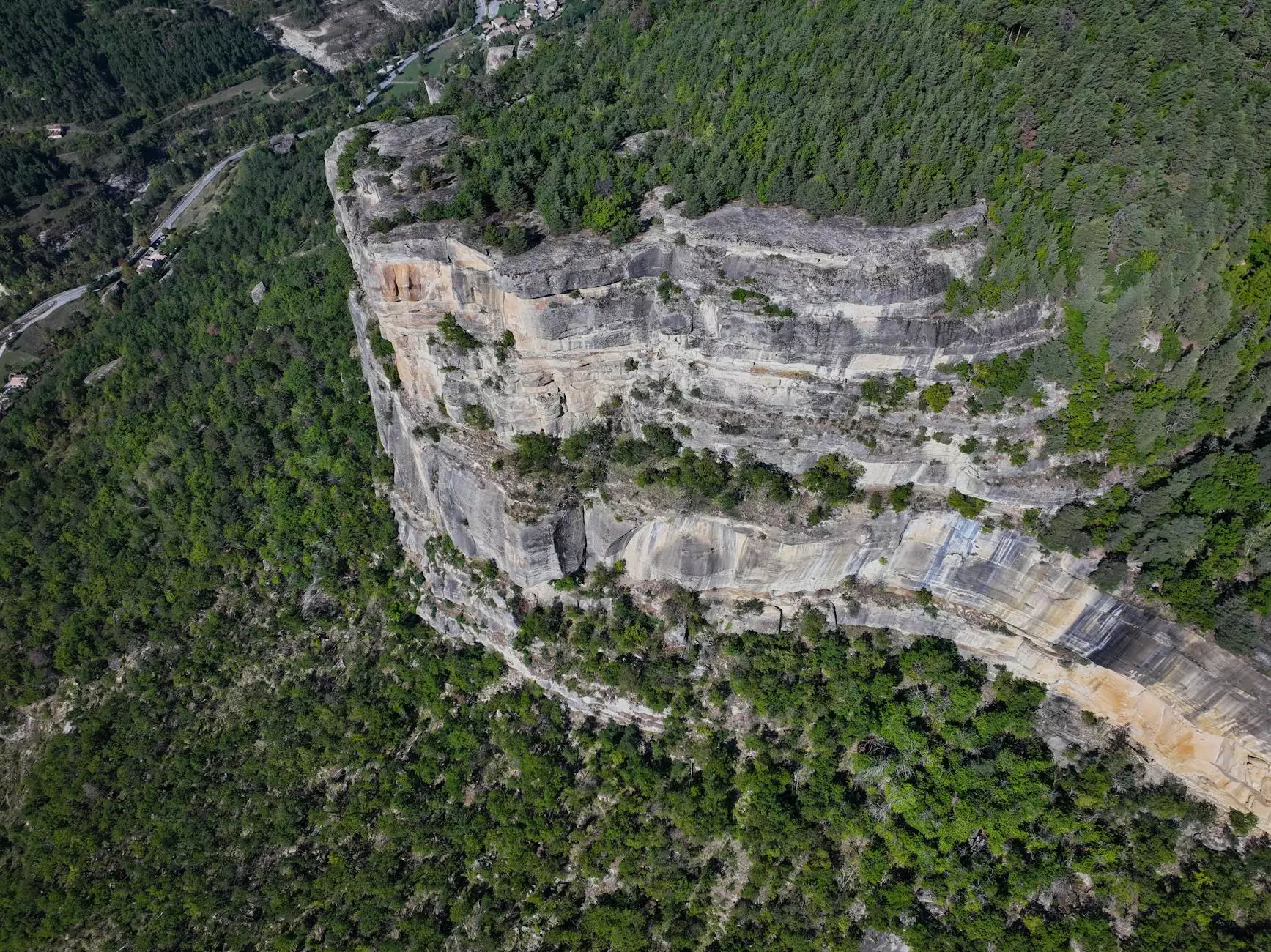
In the rapidly evolving world of artificial intelligence and machine learning, the significance of data cannot be overstated. One of the key components of developing any AI model is the quality of the data it is trained on. Among the various data types, image data is increasingly becoming prominent, leading to the need for efficient image annotation. This is where open-source image annotation tools come into play, providing businesses with scalable, cost-effective solutions to handle their data annotation needs.
The Importance of Image Annotation
Image annotation is a crucial process in preparing datasets for machine learning algorithms. The goal is to provide context and meaning to the images, which allows algorithms to learn from them effectively. The applications of image annotation are vast, including:
- Autonomous Vehicles: Annotated image data is vital for training self-driving car systems to recognize and respond to various road signs, pedestrians, and obstacles.
- Healthcare: In medical imaging, annotated datasets are used to train models in detecting diseases from X-rays, MRIs, and CT scans.
- Retail: E-commerce platforms utilize image annotation to enhance product search functionalities and improve user experience.
- Social Media: Image recognition and tagging improve content organization and user engagement within platforms.
What is an Image Annotation Tool?
An image annotation tool is a software application that enables users to add metadata to image data. Annotation can include a variety of tasks such as labeling objects in images, creating bounding boxes, segmenting images, and classifying them into defined categories. These tasks can be performed manually or automated in conjunction with machine learning algorithms.
Why Choose Open Source Annotation Tools?
Utilizing an image annotation tool open source has multiple advantages for businesses of all sizes. Let's delve into some of the most compelling reasons:
1. Cost-Effectiveness
Open source tools are free to use, which drastically reduces the cost associated with software licenses. Businesses can invest their resources into improving their data infrastructure and model accuracy rather than spending exorbitant amounts on proprietary tools.
2. Customization
With open source software, businesses have the flexibility to modify the tool to fit their unique needs. Organizations can enhance features, integrate custom workflows, and tailor the user interface, allowing for a personalized experience that proprietary tools often cannot provide.
3. Community Support
Open source projects often have active communities that support users with documentation, forums, and shared knowledge. Users can benefit from the collective experience and troubleshooting capabilities of a global user base, which can lead to improved performance and efficiency.
4. Transparency
With open source software, the code is available for anyone to inspect. This transparency means businesses can verify the security and reliability of the tool, ensuring that their data is handled appropriately without hidden vulnerabilities.
Key Features to Look for in Open Source Image Annotation Tools
When selecting an image annotation tool open source, consider the following features to ensure it meets your project requirements:
- User-Friendly Interface: A well-designed interface enhances productivity and ease of use for annotators.
- Collaboration Tools: Look for features that support teamwork and facilitate easy sharing of annotations and datasets.
- Support for Various Image Formats: The tool should accommodate multiple image types and sizes to handle various datasets.
- Export Options: Efficient exporting in different formats is crucial for seamless integration with machine learning frameworks.
- Annotation Types: Depending on your needs, the tool should support different types of annotations such as bounding boxes, polygons, or semantic segmentation.
Top Open Source Image Annotation Tools
Now that we understand the benefits of open source annotation tools, let's explore some of the leading platforms available:
1. LabelImg
LabelImg is one of the most popular open source image annotation tools that allows users to create bounding box annotations. It supports Pascal VOC and YOLO format, making it easy for users to integrate with various machine learning frameworks.
2. VGG Image Annotator (VIA)
The VGG Image Annotator provides a simple yet powerful interface for annotating images. It supports various annotation formats and is particularly useful for segmentation tasks as it allows users to draw polygons over image regions.
3. CVAT (Computer Vision Annotation Tool)
CVAT, developed by Intel, is a powerful and versatile tool that supports a wide range of annotation tasks, including image segmentation and object detection. It is designed for both individual use and team collaboration, making it a great choice for businesses.
4. Labelbox
Labelbox is another option that blends open source flexibility with a user-friendly interface. While it offers a free tier, enterprises can explore premium features that enhance collaboration and pipeline integrations.
Best Practices for Using Image Annotation Tools
To maximize the effectiveness of your image annotation tool open source, consider the following best practices:
1. Define Clear Annotation Guidelines
Having a well-defined set of annotation guidelines ensures consistency across the dataset. All annotators should be trained on what constitutes appropriate annotations, minimizing subjectivity and errors.
2. Utilize Active Learning
Incorporate active learning strategies to improve annotation quality. By periodically reviewing outputs and retraining the model on newly annotated data, you can refine your algorithms and enhance performance.
3. Leverage Collaboration Features
If your tool supports multiple users, encourage collaboration among team members. This can help distribute the workload and utilize diverse skills to enhance the annotation process.
4. Regularly Review Annotations
Conduct regular audits of annotations to ensure quality control. Peer reviews or automated quality checks can help catch errors early and improve the model's training data.
Conclusion
In conclusion, choosing an image annotation tool open source can significantly enhance your business's data annotation capabilities. With the myriad of advantages they offer, including customization, cost-effectiveness, and community support, open-source tools empower organizations to leverage their data for building robust AI applications.
The future of AI is built on high-quality datasets, and image annotation is a foundational step in this journey. By selecting the right tools and implementing best practices, businesses can make strides toward more accurate models and better solutions. Explore options like LabelImg, VIA, CVAT, and Labelbox, and unlock the potential that lies within your image data.